Atlas of immune cell infiltration in breast cancer—high M2 macrophage and low native B cellproportions are associated with poor survival
Introduction
Immunotherapy is a type of treatment that stimulates the patient’s immune system to recognize and destroy cancer cells. In recent years, immunotherapy has become of great interest in its promise to treat breast cancer (1). Some clinical trials have been performed to investigate the agents that target immune pathways in breast cancer. Lymphocyte activation gene-3 (LAG-3) antibody (IMP321) has been used to treat metastatic breast cancer (2). The cytotoxic T lymphocyte antigen-4 (CTLA-4) antibody (tremelimumab) has been used to treat metastatic hormone responsive breast cancer (3). Anti-programmed cell death protein 1 (PD-1) antibody (pembrolizumab) has been used to treat metastatic triple-negative breast cancer (4). A programmed cell death-ligand 1 (PD-L1) antagonist (MPDL3280A) has been used to treat metastatic triple-negative breast cancer (5). Most clinical studies focused on the regulation of tumor-specific T cell activation.
We have reported that the innate immune system participates in the initiation and progression of breast cancer (6,7). Knowledge of the tumor immune microenvironment is critical for developing new treatments targeting the immune system in breast cancer. In the past few years, de Melo Gagliato reported the role of lymphocyte infiltration in breast cancer prognosis and response to therapy (8). Hutloff reported that T follicular helper-like cells can drive the differentiation of B cells in breast cancer (9). Morrow et al. reported the role of gamma delta T lymphocytes in breast cancer and found that some subtypes can promote breast cancer growth and spread through immunosuppressive effects, while another subtype can suppress cancer growth through direct cytotoxicity (10). Aponte-López et al. reported the protumor and antitumor roles of mast cells in breast cancer (11). However, the complete atlas of the microenvironment of infiltrating immune cells in breast cancer has not been well investigated.
To investigate the tumor immune microenvironment, Newman et al. developed a method, CIBERSORT, to estimate the immune cell composition in a mixed cell population from cell gene expression profiles (12). Chen et al. confirmed that CIBERSORT can accurately estimate the immune composition of a tumor biopsy samples (13). CIBERSORT has been used to profile immune infiltration in early lung adenocarcinoma (14), non-small-cell lung cancer (15), microsatellite unstable colorectal cancer (16), hepatocellular carcinoma (17), and nasopharyngeal carcinoma (18). Ali et al. reported the patterns of immune infiltration in breast cancer and their clinical implications. However, their research focused on the influence of immune infiltrates on survival and treatment response. They did not provide a fundamental profile of immune cell infiltration in breast cancer (19).
The aim of this study is to illustrate the atlas of the immune cell microenvironment of breast cancer. We obtained data for breast cancer control samples from the TCGA database. The CIBERSORT algorithm was used to calculate the tumor-infiltrating immune cell (TIIC) composition. Furthermore, we performed correlation analysis of clinical information and tumor infiltrated immune cells.
We present the following article in accordance with the REMARK reporting checklist (available at https://abs.amegroups.com/article/view/10.21037/abs-20-58/rc).
Methods
Data acquisition from the cancer genome atlas (TCGA)
The gene expression profiles of breast cancer patients and normal controls were downloaded from the official TCGA website in October 2019. The expression of genes in breast invasive carcinoma (BRCA) were analyzed. Clinical characteristics from the corresponding patients were downloaded as well. The study was conducted in accordance with the Declaration of Helsinki (as revised in 2013).
Evaluation of TIICs
We used the CIBERSORT algorithm (http://cibersort.stanford.edu/) to calculate the TIIC composition (12). The TIIC immune cells included B cells, plasma cells, T cells, NK cells, macrophages, monocytes, mast cells, dendritic cells, neutrophils and eosinophils. Perl (The Perl Programming Language, version 5.28.1, http://www.perl.org) was conducted to convert IDs and group samples. The limma package of R (The R Project for Statistical Computing, version 3.5.3, https://www.r-project.org) was used to normalize the gene expression data. TIIC, P-value, root mean square error and Pearson’s correlation coefficient were quantified for each sample. Furthermore, we applied CIBERSORT to calculate the P value for the deconvolution.
Correlation analysis of clinical information and tumor infiltrated immune cells
Samples with P<0.05 were included for the subsequent clinical analysis. The clinical information matrix was merged with filtered TIIC data. The survival package of R was used to perform the survival analysis and determine the correlations between the tumor stages and immune cells. The heat map, bar plot, and violin plot were drawn by using R.
Statistical analysis
All statistical analyses were performed using R software (version 3.5.2, R Foundation). The association between clinical follow-ups and immune cells and survival curves were evaluated by log-rank test and Kaplan-Meier analysis. The correlations between TNM stage and immune cells were measured by the Wilcoxon test. Student’s t-test was used to examine the statistical relevance between two groups. All statistical tests were two-sided. P values <0.05 were considered statistically significant. The cut off value of Figures 1 and 2 is P<0.05.
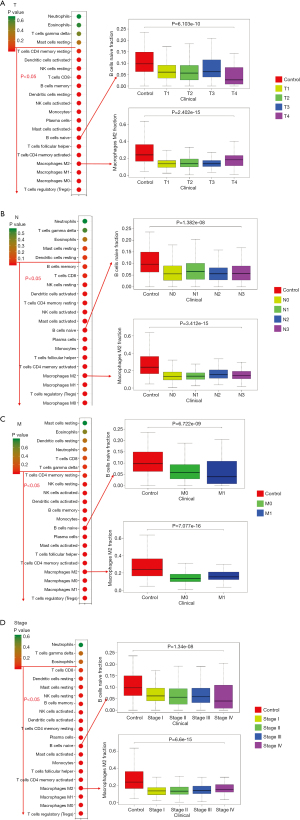
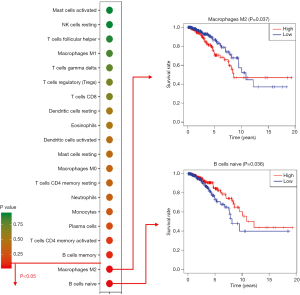
Results
Clinical characteristics
A total of 1,109 breast cancer samples and 113 control samples were downloaded for the TCGA database. After merging the clinical data with the TIIC data, 844 breast cancer samples were included in the following analysis. Detailed clinical characteristics are listed in Table 1. The average age at diagnosis was 57.57 years old.
Table 1
Clinical characteristics | Case (n=844) | (%) |
---|---|---|
TNM-T | ||
T1 | 215 | (25.47) |
T2 | 493 | (58.41) |
T2 | 106 | (12.56) |
T4 | 28 | (3.32) |
NA | 2 | (0.24) |
TNM-N | ||
N0 | 395 | (46.80) |
N1 | 297 | (35.19) |
N2 | 98 | (11.61) |
N3 | 64 | (7.58) |
NA | 11 | (1.30) |
TNM-M | ||
M0 | 709 | (84.00) |
M1 | 16 | (1.90) |
NA | 119 | (14.10) |
Stage | ||
I | 137 | (16.23) |
II | 475 | (56.28) |
III | 199 | (23.58) |
NA, not available.
Composition of TIICs: suppressed acquired immune system and activated innate immune system
We performed CIBERSORT and investigated the fractions of infiltrated immune cells between breast tumor and adjacent non-tumor tissues. A bar plot was drawn to visualize the proportions of infiltrating immune cells (Figure 3A).
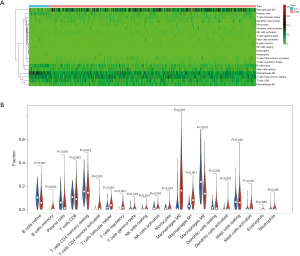
The acquired immune system was suppressed in breast cancer tissue. Compared with normal tissues, breast cancer tissues had a higher fraction of memory B cells (P=0.003), CD4 naive T cells, memory activated CD4 T cells (P<0.001), follicular helper T cells (P<0.001), and regulatory T cells (Tregs, P<0.001). Breast cancer tissues had a lower fraction of naive B cells (P<0.001), plasma cells (P<0.001) and memory resting CD4 T cells (P=0.012) than normal controls.
The innate immune system was activated in breast cancer tissue. Compared with normal tissues, breast cancer tissues had a higher fraction of resting NK cells (P=0.001), M0 macrophages (P<0.001), M1 macrophages (P<0.001), monocytes (P<0.001), activated NK cells (P=0.002), and activated mast cells (P<0.001). Breast cancer tissues had a lower fraction of M2 macrophages (P<0.001) than normal controls.
Breast cancer tissue had higher activated dendritic cell counts (P=0.001). No statistically significant differences were found in CD8 T cells (P=0.093), gamma delta T cells (P=0.378), resting dendritic cells (P=0.335), resting mast cells (P=0.945), eosinophils (P=0.882) and neutrophils (P=0.492). No significant fraction of naive CD4 T cells was found in breast cancer or normal tissues (Figure 3B).
Correlation of TIICs in breast cancer tissues
We used the corrplot package of R to draw the correlation heat map to visualize the correlations of infiltrating immune cells (Figure 4). The five immune cell types that had the highest negative correlation with M0 macrophages were monocytes, naive B cells, plasma cells, resting memory CD4 T cells and CD8 T cells. The three immune cells that were most positively correlated with M1 macrophages were CD8 T cells, activated memory CD4 T cells, and follicular helper T cells.
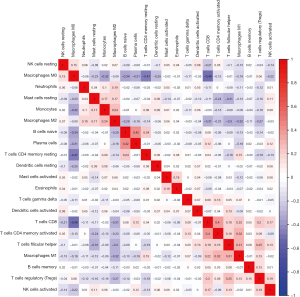
Composition of TIICs in different breast cancer subtypes
We applied CIBERSORT to investigate the fractions of infiltrated immune cells between different subtypes of breast cancer and adjacent non-tumor tissues. Triple-negative breast cancer tissue had more memory B cell and less resting mast cell infiltration than Her2+ and luminal subtype breast cancer. Luminal breast cancer had fewer activated dendritic cells than the other two subtypes (Figure 5A).
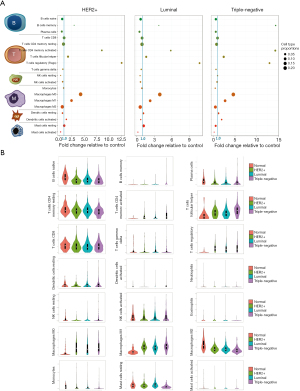
We used the vioplot package of R to draw violin plots to visualize the expression differences in infiltrating immune cells. Compared with normal tissues, the same trend was found in all three subtypes: breast cancer tissues had a higher fraction of memory B cells, CD4 naive T cells, memory activated CD4 T cells, follicular helper T cells, regulatory T cells (Tregs), resting NK cells, M0 macrophages, M1 macrophages, activated dendritic cells, and activated mast cells. Breast cancer tissues had a lower fraction of naive B cells, plasma cells, memory resting CD4 T cells, activated NK cells, monocytes, and M2 macrophages than normal controls (Figure 5B).
Correlation of the immune cells with primary tumor, lymph node and metastasis classifications and stage
We assessed the correlation of TIICs with the primary tumor, lymph node, metastasis, and stage.
The primary tumor (T) classification was associated with regulatory T cells, M0 macrophages, M1 macrophages, M2 macrophages, activated memory CD4 T cells, follicular helper T cells, naive B cells, activated mast cells, plasma cells, monocytes, activated NK cells, resting dendritic cells, memory B cells, CD8 T cells, resting NK cells, activated dendritic cells, and resting memory CD4 T cells (Figure 1A).
The lymph node (N) classification was associated with M0 macrophages, regulatory T cells, M1 macrophages, M2 macrophages, activated memory CD4 T cells, follicular helper T cells, monocytes, plasma cells, naive B cells, activated mast cells, activated NK cells, resting memory CD4 T cells, activated Dendritic cells, resting NK cells, CD8 T cells, and memory B cells (Figure 1B).
The metastasis (M) classification was associated with regulatory T cells, M1 macrophages, M0 macrophages, M2 macrophages, activated memory CD4T cells, follicular helper T cells, activated mast cells, plasma cells, naive B cells, monocytes, memory B cells, activated dendritic cells, activated NK cells, resting NK cells, and memory resting CD4 T cells (Figure 1C).
Tumor stage was associated with regulatory T cells, M0 macrophages, M1 macrophages, M2 macrophages, memory-activated CD4 T cells, follicular helper T cells, monocytes, activated mast cells, resting memory CD4 T cells, activated dendritic cells, activated NK cells, resting NK cells, resting mast cells, and resting dendritic cells (Figure 1D).
High M2 macrophage and low native B cell counts were associated with poor survival
Furthermore, we investigated the correlation of immune cells with survival rate. M2 macrophages and naive B cells could predict the survival rate. M2 macrophages are a risk factor for breast cancer. A low fraction of M2 macrophages was associated with a high survival rate (P=0.037). Naive B cells are protective factors. A high fraction of naive B cells was associated with a high survival rate (P=0.036) (Figure 2).
Discussion
In this study, we illustrated an atlas of the immune cell microenvironment of breast cancer. Our findings suggest that the adaptive immune system is suppressed, while the innate immune system is activated in breast cancer tissue. The same trends were found in all three (Her2+, luminal and triple-negative) subtypes. Furthermore, we found that high M2 macrophage and low native B cell counts were associated with poor survival.
The adaptive immune system, also known as the acquired immune system, was suppressed in breast cancer tissues. Adaptive immunity provides an antigen-specific response following exposure to a microbial pathogen or antigen. The adaptive immune system mainly involves B cells and T cells. B cells were suppressed in this study, as breast cancer tissue had a low fraction of plasma cells and a high fraction of memory B cells. Most previous studies supported that the infiltration of plasma cells (activated B cells) could predict better survival (20,21). Furthermore, regulatory T cells, which can suppress antitumor immunity, had a high fraction in breast cancer tissue. Plitas et al. found that regulatory T cells contribute to cancer progression (22,23). High regulatory T cells suppress the local antitumor immune reaction. Moreover, although not statistically significant, CD8+ T cells generally had a low fraction in breast cancer tissue. Savas et al. reported that CD8+ T cells contribute to improved patient survival in early-stage triple-negative breast cancer (24). Huang et al. reported that CD8+ T cells mediate antitumor immunity (25). Our findings suggested that breast cancer cells suppress the human adaptive immune system.
The innate immune system was activated in breast cancer tissue. Innate immunity refers to the nonspecific defense against tumors. Tumoricidal M1 macrophages, monocytes, and activated mast cells were present at higher fractions in breast cancer tissues. M1macrophages have proinflammatory and anticancer functions. A high number of M1 macrophages was significantly associated with improved breast cancer survival (26). Human monocytes predicted a better survival rate (27). The role of mast cells in breast cancer is complex. It may exert both protumor and antitumor effects. Furthermore, tumor-promoting M2 macrophages were present at a lower fraction in breast cancer tissues. M2 macrophages are immunosuppressive and contribute to matrix remodeling, hence favoring tumor growth. Sousa et al. found that high numbers of M2 macrophages were strongly associated with poor differentiation, fast proliferation and estrogen receptor negativity in primary breast tumors (28). Our results supported that breast cancer activated the innate immune system.
Antigen-presenting cells (APC) were activated in breast cancer tissue. APCs can present antigen to appropriate T cell receptors. In this study, we found that breast cancer tissue had a high fraction of activated dendritic cells. Dendritic cells are necessary for T cell-mediated cancer immunity (29). Our findings suggested that the suppression of the adaptive immune system does not occur through the suppression of APCs.
A high M2 macrophage fraction was associated with poor survival is this study. M2 macrophages are alternatively activated macrophages that are associated with tissue repair and wound healing. M2 macrophages have tumor-promoting effect in many cancers (30). Increased macrophage infiltration is associated with poor prognosis in breast, cervical, lung, thyroid, hepatocellular, and ovarian cancers (30). Inhibition of M2 macrophage differentiation suppressed established tumors and increased survival (31). However, an interesting finding is that the proportion of M2 macrophage is lower in all three sub-types and in all four stages of tumor samples compared with control samples. This may depend on the microenvironmental signals. Macrophages are polarized into M1 macrophages or M2 macrophages in different microenvironments. Based on our study, it seems that the microenvironment in breast cancer tissue triggers macrophages polarize into M1, instead of M2. That’s why tumoricidal M1 macrophages were present at higher fractions in breast cancer tissues, while tumor-promoting M2 macrophages were present at a lower fraction in breast cancer tissues. Another interesting finding of M2 macrophages is that the lines of red and blue are crossing in survival analysis. The worse outcome is observed in low M2 infiltrated tumor group after about 10 years. One possibility is that the sample size is low after 10 years follow up. Another possibility is M2 macrophages may play complex roles in breast cancer microenvironment.
A low fraction of naive B cells was associated with poor survival in the present study. Naive B cells are a type of B cells that have not been exposed to any antigen. A naive B cell may become a plasma cell or a memory B cell once exposed to an antigen. A high fraction of naive B cells suggests few activated B cells. Activated B cell infiltration predicts better response to therapy and longer survival (32).
In conclusion, breast cancer tissues suppress the adaptive immune system and activate the innate immune system (Figure 6). High M2 macrophage and low native B cell counts are associated with poor survival.
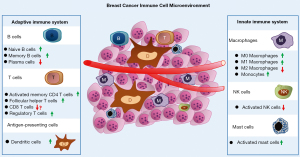
Acknowledgments
We thank the TCGA working group for generating publicly available data.
Funding: None.
Footnote
Reporting Checklist: The authors have completed the REMARK reporting checklist. Available at https://abs.amegroups.com/article/view/10.21037/abs-20-58/rc
Conflicts of Interest: All authors have completed the ICMJE uniform disclosure form (available at https://abs.amegroups.com/article/view/10.21037/abs-20-58/coif). ZMS serves as the Honorary Editor-in-Chief of Annals of Breast Surgery from September 2017 to August 2022. The other authors have no conflicts of interest to declare.
Ethical Statement: The authors are accountable for all aspects of the work in ensuring that questions related to the accuracy or integrity of any part of the work are appropriately investigated and resolved. The study was conducted in accordance with the Declaration of Helsinki (as revised in 2013).
Open Access Statement: This is an Open Access article distributed in accordance with the Creative Commons Attribution-NonCommercial-NoDerivs 4.0 International License (CC BY-NC-ND 4.0), which permits the non-commercial replication and distribution of the article with the strict proviso that no changes or edits are made and the original work is properly cited (including links to both the formal publication through the relevant DOI and the license). See: https://creativecommons.org/licenses/by-nc-nd/4.0/.
References
- Yang Y. Cancer immunotherapy: harnessing the immune system to battle cancer. J Clin Invest 2015;125:3335-7. [Crossref] [PubMed]
- Perez-Santos M, Anaya-Ruiz M, Cebada J, et al. LAG-3 antagonists by cancer treatment: a patent review. Expert Opin Ther Pat 2019;29:643-51. [Crossref] [PubMed]
- Khunger A, Rytlewski JA, Fields P, et al. The impact of CTLA-4 blockade and interferon-alpha on clonality of T-cell repertoire in the tumor microenvironment and peripheral blood of metastatic melanoma patients. Oncoimmunology 2019;8:e1652538. [Crossref] [PubMed]
- Geoerger B, Kang HJ, Yalon-Oren M, et al. Pembrolizumab in paediatric patients with advanced melanoma or a PD-L1-positive, advanced, relapsed, or refractory solid tumour or lymphoma (KEYNOTE-051): interim analysis of an open-label, single-arm, phase 1-2 trial. Lancet Oncol 2020;21:121-33. [Crossref] [PubMed]
- Geoerger B, Zwaan CM, Marshall LV, et al. Atezolizumab for children and young adults with previously treated solid tumours, non-Hodgkin lymphoma, and Hodgkin lymphoma (iMATRIX): a multicentre phase 1-2 study. Lancet Oncol 2020;21:134-44. [Crossref] [PubMed]
- Fan L, Zhou P, Hong Q, et al. Toll-like receptor 3 acts as a suppressor gene in breast cancer initiation and progression: a two-stage association study and functional investigation. Oncoimmunology 2019;8:e1593801. [Crossref] [PubMed]
- Fan L, Zhou P, Chen AX, et al. Toll-like receptor 3 -926T>A increased the risk of breast cancer through decreased transcriptional activity. Oncoimmunology 2019;8:e1673126. [Crossref] [PubMed]
- de Melo Gagliato D, Cortes J, Curigliano G, et al. Tumor-infiltrating lymphocytes in Breast Cancer and implications for clinical practice. Biochim Biophys Acta Rev Cancer 2017;1868:527-37. [Crossref] [PubMed]
- Hutloff A. T Follicular Helper-Like Cells in Inflamed Non-Lymphoid Tissues. Front Immunol 2018;9:1707. [Crossref] [PubMed]
- Morrow ES, Roseweir A, Edwards J. The role of gamma delta T lymphocytes in breast cancer: a review. Transl Res 2019;203:88-96. [Crossref] [PubMed]
- Aponte-López A, Fuentes-Panana EM, Cortes-Munoz D, et al. Mast Cell, the Neglected Member of the Tumor Microenvironment: Role in Breast Cancer. J Immunol Res 2018;2018:2584243. [Crossref] [PubMed]
- Newman AM, Liu CL, Green MR, et al. Robust enumeration of cell subsets from tissue expression profiles. Nat Methods 2015;12:453-7. [Crossref] [PubMed]
- Chen B, Khodadoust MS, Liu CL, et al. Profiling Tumor Infiltrating Immune Cells with CIBERSORT. Methods Mol Biol 2018;1711:243-59. [Crossref] [PubMed]
- Kurbatov V, Balayev A, Saffarzadeh A, et al. Digital Inference of Immune Microenvironment Reveals "Low Risk" Subtype of Early Lung Adenocarcinoma. Ann Thorac Surg 2020;109:343-9. [Crossref] [PubMed]
- Chen F, Yang Y, Zhao Y, et al. Immune Infiltration Profiling in Nonsmall Cell Lung Cancer and Their Clinical Significance: Study Based on Gene Expression Measurements. DNA Cell Biol 2019;38:1387-401. [Crossref] [PubMed]
- Narayanan S, Kawaguchi T, Peng X, et al. Tumor Infiltrating Lymphocytes and Macrophages Improve Survival in Microsatellite Unstable Colorectal Cancer. Sci Rep 2019;9:13455. [Crossref] [PubMed]
- Li W, Xu L, Han J, et al. Identification and Validation of Tumor Stromal Immunotype in Patients With Hepatocellular Carcinoma. Front Oncol 2019;9:664. [Crossref] [PubMed]
- Luo MS, Huang GJ, Liu BX. Immune infiltration in nasopharyngeal carcinoma based on gene expression. Medicine (Baltimore) 2019;98:e17311. [Crossref] [PubMed]
- Ali HR, Chlon L, Pharoah PD, Markowetz F, Caldas C. Patterns of Immune Infiltration in Breast Cancer and Their Clinical Implications: A Gene-Expression-Based Retrospective Study. PLoS Med 2016;13:e1002194. [Crossref] [PubMed]
- Zhang Z, Zhu Y, Wang Z, et al. Yin-yang effect of tumor infiltrating B cells in breast cancer: From mechanism to immunotherapy. Cancer Lett 2017;393:1-7. [Crossref] [PubMed]
- Hollern DP, Xu N, Thennavan A, et al. B Cells and T Follicular Helper Cells Mediate Response to Checkpoint Inhibitors in High Mutation Burden Mouse Models of Breast Cancer. Cell 2019;179:1191-206.e21. [Crossref] [PubMed]
- Plitas G, Konopacki C, Wu K, et al. Regulatory T Cells Exhibit Distinct Features in Human Breast Cancer. Immunity 2016;45:1122-34. [Crossref] [PubMed]
- Speiser DE, Ho PC, Verdeil G. Regulatory circuits of T cell function in cancer. Nat Rev Immunol 2016;16:599-611. [Crossref] [PubMed]
- Savas P, Virassamy B, Ye C, et al. Single-cell profiling of breast cancer T cells reveals a tissue-resident memory subset associated with improved prognosis. Nat Med 2018;24:986-93. [Crossref] [PubMed]
- Huang Y, Ma C, Zhang Q, et al. CD4+ and CD8+ T cells have opposing roles in breast cancer progression and outcome. Oncotarget 2015;6:17462-78. [Crossref] [PubMed]
- Honkanen TJ, Tikkanen A, Karihtala P, et al. Prognostic and predictive role of tumour-associated macrophages in HER2 positive breast cancer. Sci Rep 2019;9:10961. [Crossref] [PubMed]
- Allaoui R, Bergenfelz C, Mohlin S, et al. Cancer-associated fibroblast-secreted CXCL16 attracts monocytes to promote stroma activation in triple-negative breast cancers. Nat Commun 2016;7:13050. [Crossref] [PubMed]
- Sousa S, Brion R, Lintunen M, et al. Human breast cancer cells educate macrophages toward the M2 activation status. Breast Cancer Res 2015;17:101. [Crossref] [PubMed]
- Gardner A, Ruffell B. Dendritic Cells and Cancer Immunity. Trends Immunol 2016;37:855-65. [Crossref] [PubMed]
- Ding L, Liang G, Yao Z, et al. Metformin prevents cancer metastasis by inhibiting M2-like polarization of tumor associated macrophages. Oncotarget 2015;6:36441-55. [Crossref] [PubMed]
- Pyonteck SM, Akkari L, Schuhmacher AJ, et al. CSF-1R inhibition alters macrophage polarization and blocks glioma progression. Nat Med 2013;19:1264-72. [Crossref] [PubMed]
- Garaud S, Zayakin P, Buisseret L, et al. Antigen Specificity and Clinical Significance of IgG and IgA Autoantibodies Produced in situ by Tumor-Infiltrating B Cells in Breast Cancer. Front Immunol 2018;9:2660. [Crossref] [PubMed]
Cite this article as: Fan L, Ma LX, Zhou P, Shao ZM. Atlas of immune cell infiltration in breast cancer—high M2 macrophage and low native B cellproportions are associated with poor survival. Ann Breast Surg 2022;6:3.