Area deprivation, machine learning, and breast cancer surgery
Receipt of timely surgery for women with breast cancer is critical to survival. Labilloy et al. investigated factors associated with non-adherence to timely surgery among women who were diagnosed with stage 0–III breast cancer and were treated at the University of Florida Health Hospital in Jacksonville, FL, USA based on data from electronic health records and the hospital’s tumor registry (1). From the results of machine learning models, the authors concluded that area deprivation index (ADI) was the most important variable among demographic (race and age), clinical (stage at diagnosis and type of surgery), and socioeconomic (insurance type and ADI) risk factors in predicting non-adherence to timely surgery.
Multiple studies have uncovered that socioeconomic status (SES) might have surpassed demographic and clinical risk factors in determining the receipt of surgery among women with breast cancer (2,3). The results from Labilloy et al.’s study reinforced these findings by using ADI, a composite index of SES calculated from 17 census variables covering domains of education, employment, income, housing (costs and crowding), and transportation access (4) at the block group level which generally contains between 600 and 3,000 people. In their study, ADI was classified as high (top 40%, or more deprived) vs. low (bottom 60%, or less deprived) among all block groups in Florida, indicating a rather crude surrogate for individual-level SES. Nevertheless, ADI was still identified as the most important variable in predicting non-adherence to timely breast cancer surgery. Recent studies also identified strong associations between ADI and breast cancer screening (5), late-stage diagnosis (6), and survival (7) in larger US populations. Findings from these studies suggest that women with breast cancer living in deprived areas with low SES might have been disadvantaged at all segments along the breast cancer care continuum, from prevention and screening to diagnosis, treatment, and survivorship care.
As discussed, ADI has been shown to be an effective indicator of SES in its association with breast cancer outcomes. As a composite index, ADI has the advantage of reflecting the multidimensional characteristics of a community’s socioeconomic position (4). However, it is unclear which dimensions of SES in ADI played a key role in these associations. This limits the value of ADI for researchers and practitioners who aim to design interventions to improve inequities in breast cancer care.
One approach to mitigate this limitation of ADI is to include non-composite SES variables in tree-based ensemble machine learning models such as the AdaBoost and random forest algorithms. An advantage of these models is that they can incorporate many predictors without concerns about correlations between them, or the multicollinearity problem, leading to less reliable statistical inferences as often found in conventional statistical methods. By including additional SES variables as predictors, we would know which SES variables are more important than others, including ADI, from the variable importance measure.
One caveat of the tree-based ensemble models of AdaBoost and random forest is that they act like a black box, where we cannot capture traditional statistical measures such as coefficient, odds ratio, and even direction of the association (i.e., whether a predictor is positively or negatively associated with the outcome). To mitigate this limitation, one can use the direction of association from the Pearson’s correlation coefficient in combination with the variable importance measure from the machine learning model, such as the one presented in Figure 1 in predicting early-onset colorectal cancer incidence rate in a recent study using random forest analysis (8).
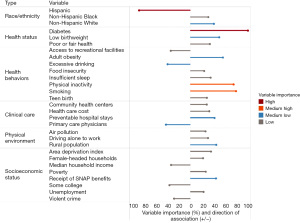
A recent study adopted a novel approach to identifying county phenotypes of late-stage breast cancer (LSBC) by using the classification and regression tree (CART) algorithm (6). In Figure 2, each path down to a terminal node represents a phenotype of LSBC. For example, with the highest risk of LSBC (median percentage of LSBC: 40.1%), phenotype 7 was characterized as counties having more uninsured middle-aged women (>11.6%), a greater area deprivation (ADI >99.7), and more people under poverty (>26.1%). In contrast, the lowest risk phenotype of LSBC (phenotype 1, median percentage of LSBC: 30.6%) can be characterized as counties having fewer uninsured middle-aged women (≤11.6%) and a higher rate of screening mammography (>68.1%). This CART approach not only identifies important variables, but also classifies observations according to their distinct phenotypes, or combination of characteristics associated with LSBC. This phenotype approach was also adopted in other health outcomes including premature cardiovascular disease (9) and self-reported fair/poor health status (10).
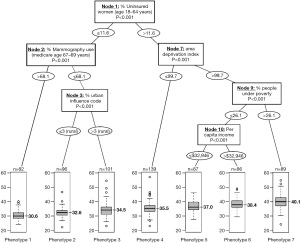
In summary, by using novel machine learning approaches, Labilloy et al.’s study suggests that the community-level ADI was the most important variable in predicting non-adherence to timely breast cancer surgery when individual-level demographic and clinical factors were also considered (1). While medical care is estimated to account for only 10–20% of the modifiable contributors to health outcomes for a population (11), Labilloy et al. demonstrate that social determinants of health (SDOH) also play a key role in the receipt of medical care, suggesting that SDOH and medical care influence the health of our population interactively. This study can be improved, however, by including non-composite SES variables in the machine learning models and by using an alternative approach to identify phenotypes of non-adherence to timely breast cancer surgery using CART analysis, as mentioned above. Future studies should consider using individual-level SDOH, such as data from the data analytics platform LexisNexis (12), to better understand the mechanisms at play in cancer outcomes.
Acknowledgments
Funding: None.
Footnote
Provenance and Peer Review: This article was commissioned by the editorial office, Annals of Breast Surgery. The article did not undergo external peer review.
Conflicts of Interest: Both authors have completed the ICMJE uniform disclosure form (available at https://abs.amegroups.com/article/view/10.21037/abs-23-12/coif). W.D. is supported by grants from the National Cancer Institute, Case Comprehensive Cancer Center (P30 CA043703), the National Institutes of Health (R15 NR017792 and UH3-DE025487), the American Cancer Society (RWIA-20-111-02 RWIA and 132678-RSGI-19-213-01-CPHPS), and having contracts from Cleveland Clinic Foundation, including a subcontract from Celgene Corporation, outside the submitted work. S.L. has no conflicts of interest to declare.
Ethical Statement: The authors are accountable for all aspects of the work to ensure that questions related to the accuracy or integrity of any part of the work are appropriately investigated and resolved.
Open Access Statement: This is an Open Access article distributed in accordance with the Creative Commons Attribution-NonCommercial-NoDerivs 4.0 International License (CC BY-NC-ND 4.0), which permits the non-commercial replication and distribution of the article with the strict proviso that no changes or edits are made and the original work is properly cited (including links to both the formal publication through the relevant DOI and the license). See: https://creativecommons.org/licenses/by-nc-nd/4.0/.
References
- Labilloy G, Jasra B, Widrich J, et al. Machine learning determined risk factors associated with non-adherence to timely surgery for breast cancer patients. Ann Breast Surg 2024;8:3.
- Rose J, Oliver Y, Sage P, et al. Factors affecting timely breast cancer treatment among black women in a high-risk urban community: a qualitative study. BMC Womens Health 2022;22:354. [Crossref] [PubMed]
- Obeng-Gyasi S, Rose J, Dong W, et al. Is Medicaid Expansion Narrowing Gaps in Surgical Disparities for Low-Income Breast Cancer Patients? Ann Surg Oncol 2022;29:1763-9. [Crossref] [PubMed]
- Singh GK. Area deprivation and widening inequalities in US mortality, 1969-1998. Am J Public Health 2003;93:1137-43. [Crossref] [PubMed]
- Anderson RT, Yang TC, Matthews SA, et al. Breast cancer screening, area deprivation, and later-stage breast cancer in Appalachia: does geography matter? Health Serv Res 2014;49:546-67. [Crossref] [PubMed]
- Dong W, Bensken WP, Kim U, et al. Phenotype Discovery and Geographic Disparities of Late-Stage Breast Cancer Diagnosis across U.S. Counties: A Machine Learning Approach. Cancer Epidemiol Biomarkers Prev 2022;31:66-76. [Crossref] [PubMed]
- Luningham JM, Seth G, Saini G, et al. Association of Race and Area Deprivation With Breast Cancer Survival Among Black and White Women in the State of Georgia. JAMA Netw Open 2022;5:e2238183. [Crossref] [PubMed]
- Dong W, Kim U, Rose J, et al. Geographic Variation and Risk Factor Association of Early Versus Late Onset Colorectal Cancer. Cancers (Basel) 2023;15:1006. [Crossref] [PubMed]
- Dong W, Motairek I, Nasir K, et al. Risk factors and geographic disparities in premature cardiovascular mortality in US counties: a machine learning approach. Sci Rep 2023;13:2978. [Crossref] [PubMed]
- Koroukian SM, Schiltz N, Warner DF, et al. Combinations of Chronic Conditions, Functional Limitations, and Geriatric Syndromes that Predict Health Outcomes. J Gen Intern Med 2016;31:630-7. [Crossref] [PubMed]
- Hood CM, Gennuso KP, Swain GR, et al. County Health Rankings: Relationships Between Determinant Factors and Health Outcomes. Am J Prev Med 2016;50:129-35. [Crossref] [PubMed]
- Stinchcomb DG, Roeser A. NCI/SEER Residential History Project. 2016. Available online: https://www.westat.com/sites/default/files/NCISAS/NCI_Res_Hist_Proj_Tech_Rpt_v2sec.pdf
Cite this article as: Dong W, Li S. Area deprivation, machine learning, and breast cancer surgery. Ann Breast Surg 2024;8:2.